By Erica Yee • August 2, 2018
To invent the first commercially viable electric light bulb, Thomas Edison and his assistants tested thousands of materials to use for the filament until they found one that lasted long enough. This traditional “Edisonian” trial-and-error process of materials discovery is still fundamentally how we design materials almost 150 years later. However, through a method called “materials by design,” researchers can now avoid many of the expensive dead ends that slowed Edison down.
Since launching in 2011, the Materials Project, a Department of Energy program based at Lawrence Berkeley National Laboratory (Berkeley Lab), has been on the forefront of this revolution with a giant, searchable repository of data available to the whole materials science community . Using computational materials science, which integrates supercomputers, advanced mathematics, and quantum mechanics, researchers can virtually simulate thousands of compounds every day to find the best candidates to test in the laboratory. They use a growing database of 80,000 inorganic compounds to select existing materials and create novel combinations for given applications.
“We’re far more efficient because materials problems and materials challenges underpin everything we do in science, engineering, and technology,” said Shyam Dwaraknath, a materials research scientist in Berkeley Lab’s Applied Energy Materials Group under Materials Project co-founder and director Kristin Persson.
Dwaraknath’s role is to build computational capabilities for the Materials Project that can target a variety of materials challenges. His work addresses questions such as how to design materials that are lighter, stronger, cheaper to produce, easier to make, and less energy consuming in the production process. Applications range from the battery material for the next best car to replacements for the lead-based materials often used in gas stove lighters.
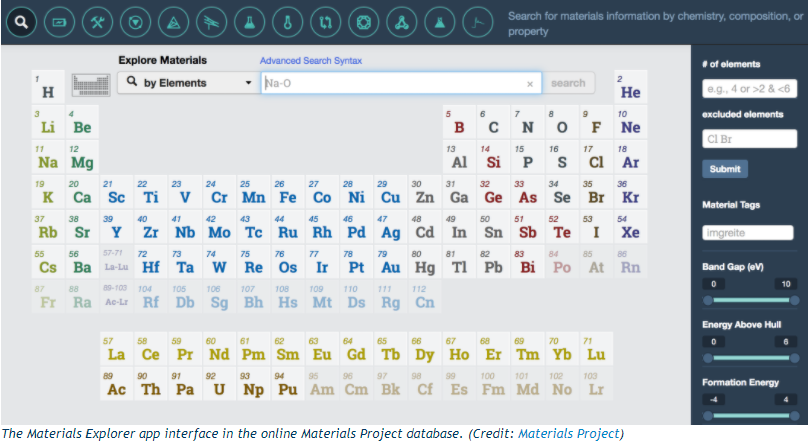
Calculating constantly around the country
The scale of the calculations required to solve these kinds of problems is vast. Materials scientists explore both “horizontally” in terms of properties of interest and “vertically” as informed by the chemistry of specific materials.
“There are nearly an infinite number of combinations of atoms. So regardless of which way you go, you can compute until there’s no more computation time,” said Dwaraknath.
To accommodate the Materials Project’s constant stream of calculations, researchers tap into a variety of supercomputing resources. In addition to the National Energy Research Scientific Computing Center (NERSC), the national scientific computing facility based at Berkeley Lab that hosts the project database, the group and its collaborators have access to computing facilities at Oak Ridge and Argonne national laboratories. They also rely heavily on shared institutional computing clusters: Lawrencium, managed by Berkeley Lab’s Scientific Computing Group, and Savio, managed by Berkeley Research Computing at UC Berkeley. In 2017, Materials Project researchers used hundreds of millions of CPU hours.
“Our infrastructure is a generic computing workflow that we can deploy on all these supercomputers,” said Dwaraknath. “Depending on the type of resource available and the priorities for our projects, we deploy calculations at the different facilities.”
Using known materials to drive parameters
Much of the work in the Materials Project revolves around using known materials to inform novel new ones. A classic example of driving parameters this way is with diamond, a material researchers struggle to create synthetically but is formed naturally under extreme temperature and pressure deep in the Earth’s mantle. Scientists can measure diamond’s qualities, such as hardness, that are associated with its physical structure. Then they can seek other materials that have similar structure but are easier to synthesize. One such substance is silicon carbide, which is used to form a ceramic material used in brakes because of its ability to withstand high temperatures.
“That’s a material we can compute and show has similar properties of diamond,” said Dwaraknath. “It’s much easier to make because of the chemistry that drives it rather than the physical parameters of the temperature and pressure to create that material.”
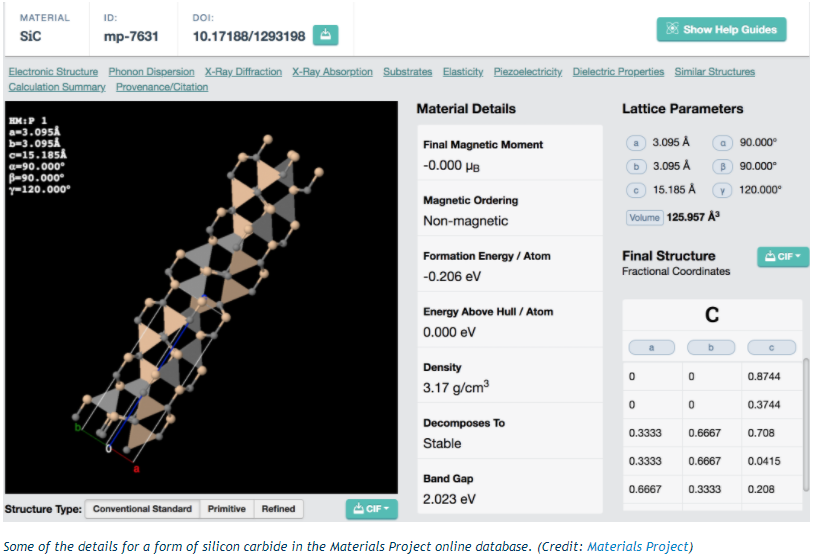
Cutting to the chase with a “synthesizability skyline”
Computational materials scientists can explore thousands of combinations of a material in the course of a day using high-performance computing.
“You can think of the computer as a virtual lab where I can do the same processes, but at a more rapid pace. I can get results very quickly. I can fail fast so I can try over and over and over again until I find that one combination that is going to work well,” said Dwaraknath.
However, a major bottleneck to taking a theoretical discovery and making an impact with it is producing real-world materials in a lab. Experimental scientists work much more slowly when translating virtual candidates from computed results into applications. While computationalists can prescribe exactly where the atoms are in a model, experimentalists have imperfect control over materials. Additionally, when materials scientists predict a material, they don’t always know how to predict the best method by which to create it. Computational materials scientists are addressing these challenges by building models to expedite experimental processes so scientists in the lab can narrow down which combinations to attempt.
Dwaraknath is one of the authors on a Berkeley Lab study published recently in Science Advances that showed how to use the data and computational powers of the Materials Project to create a “synthesizability skyline.” Instead of trying to determine which materials can be made, they went after the opposite problem: which materials cannot be made. By comparing energies of the crystalline (ordered structured) and amorphous (more randomly structured) phases of materials, they were able to calculate limits on a comparable energy scale. Any structure with an energy above the threshold cannot be made because the atoms in the entity would fall apart. There’s no guarantee that a structure under the threshold can be made, but experimentalists can discard everything they know cannot be made.
“The reason we call it a skyline is because it encompasses all materials,” said Dwaraknath. “The methodology is generic, and we did it on a variety of materials systems that are very important for any modern technology.”
Because the researchers had enough materials science data on a large scale, they figured out this process without having to physically try synthesizing materials. They were able to validate that the calculated energy window accurately reflects the threshold observed in the cases of known materials that have been produced naturally.
“In every single situation, regardless of the chemistry and the structures that are available, we got there,” he continued. “We never cut off materials that are known to be made, including several materials that can only be made in very harsh conditions.”
Rather than relying on intuition and rules of thumb, which may lead to arbitrarily discarding potentially useful materials or false positives, experimental scientists can use this calculated limit to identify the narrowest energy range for successful synthesis. The discovery has the potential to bridge the gap from predicting materials to synthesizing them, accelerating materials discovery for wide-ranging applications such as batteries, structural materials, and solar materials.
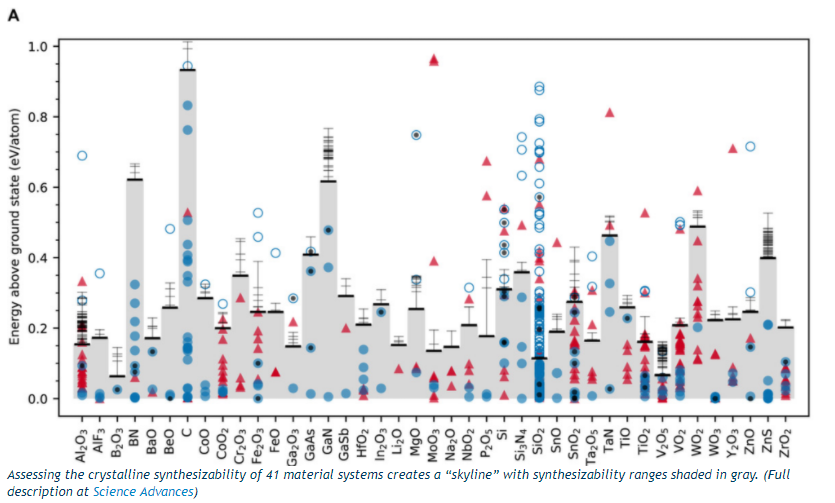
Teaching computers to see materials like a scientist
Looking ahead, the Materials Project is integrating machine learning in order to get a computer to “see” (model) materials and molecules the way a human does.
“In materials science, one of the biggest problems is: How do you take a material and describe it in a way in which a machine-learning algorithm can accurately represent and take apart all the important aspects of it?” explained Dwaraknath.
The visualization space can be complicated with molecules of different sizes and information densities. For example, while graphite could be depicted with two atoms, there might be a different material that needs 100 atoms to capture its intricacies. The challenge lies in presenting both at scale using mathematical representations of their images from Materials Project data.
Every part of the group’s research grows the database, either directly or for an application.
“There’s a synergy in that there’s so much left to be done that no matter where you go and try to look, there’s something you can explore to find a new material for your application, but also adds to the Materials Project because computational materials science is an infant field in comparison to most other sciences,” said Dwaraknath.